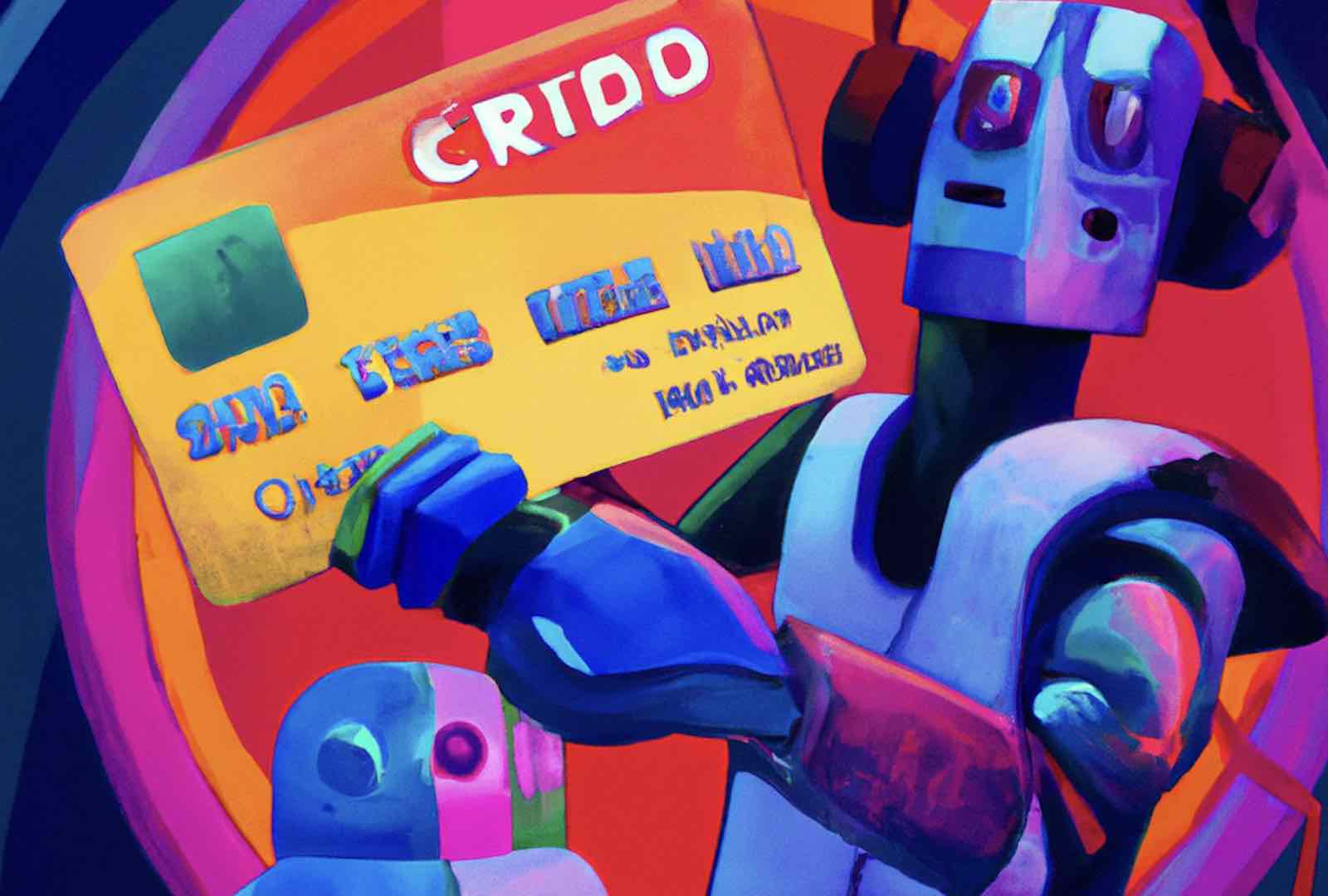
Correctly categorizing credit card transactions is difficult, but crucial for understanding your expenses.
For example:
- Is it obvious WalMart CC DES:WM EPAY ID:1738284414 INDN: 6032203774694367 CO ID:9069872103 WEB is a monthly payment on a Walmart credit card, not a purchase at a Walmart store, and should be classified as a Loan / Credit Card Payment?
- How could your ML system detect that WEED MAN 309-8279390 IL is a payment for lawn services (classified as General Services - Home Repair + Maintenance), not a marijuana purchase?
- If you don’t use labelers familiar with US culture, would they know that DUNKIN #343461 PLYMOUTH /MA US CARD PURCHASE refers to Dunkin’ Donuts, and classify it as Food & Drink?
To help you (or your favorite fintech company!) train better financial transaction classification models, we built a free dataset of credit card and debit transactions, labeled with the expense category and the original purchaser’s intent. Explore the dataset or download it here!
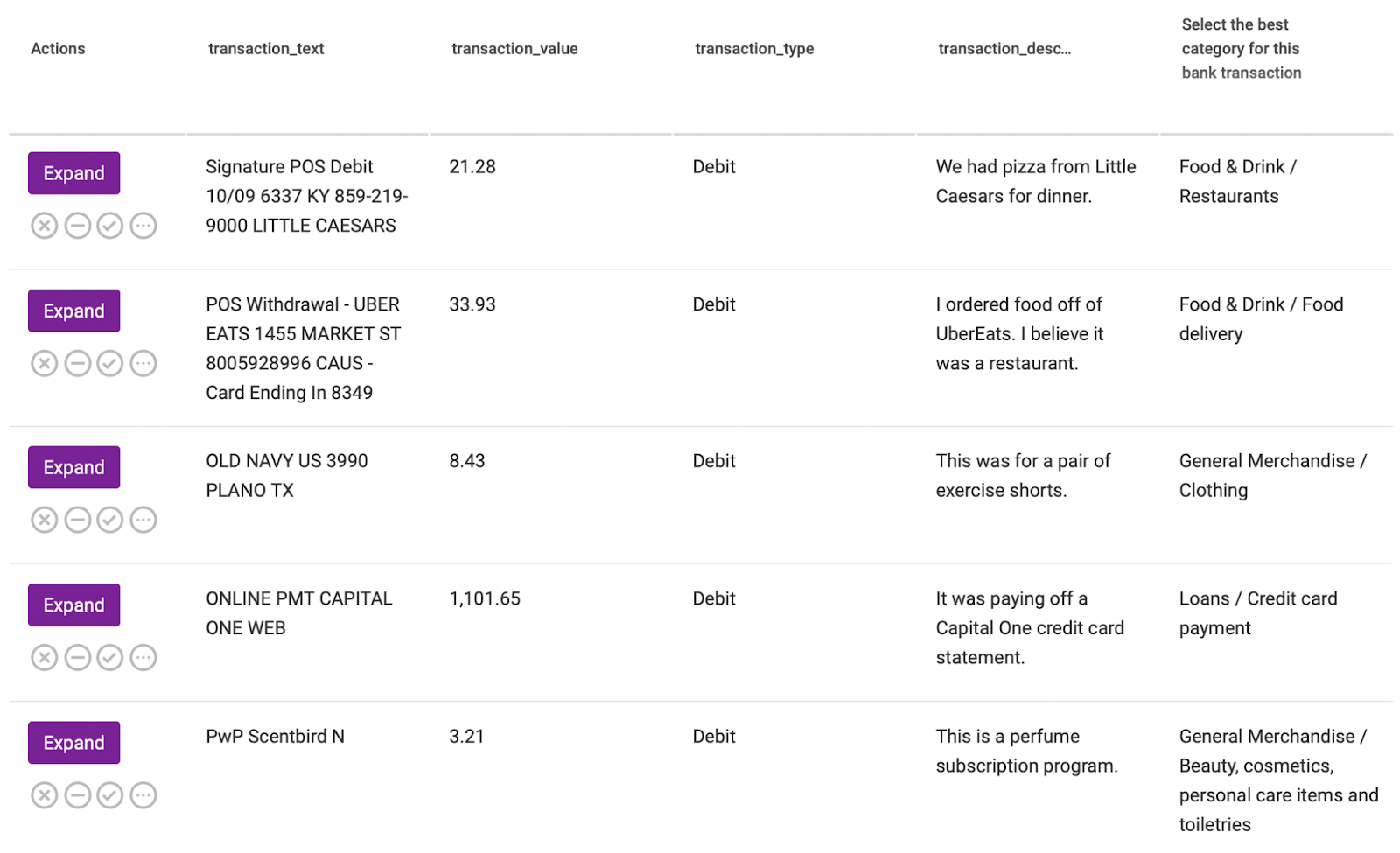
The Financial Transactions Dataset
To form this dataset, we first asked Surgers to collect their historical credit card and debit transactions. For each transaction, they gathered the following information:
- Transaction Text
- Transaction Value
- Transaction Type (Credit or Debit)
They then annotated each transaction with two fields:
- A freeform description of the purchase
- Expense category
Here are a few examples. Explore the full dataset on our platform!
Example #1
Transaction Text: HOLLYWOOD BOWL CD 3041
Transaction Value: $19.97
Transaction Type: Debit
Transaction Description: Money spent at a bowling alley during a family outing.
Expense Category: Entertainment
Example #2
Transaction Text: Einsteinmobileapp
Transaction Value: $4.70
Transaction Type: Debit
Transaction Description: This is a breakfast place named Einstein Bros Bagels. It was an order through their mobile app.
Expense Category: Food & Drink - Restaurants
How We Labeled It
Data Labeling Workforce
Labeling financial transactions can be surprisingly tricky. In order to do a good job, you need to understand esoteric financial transaction formats, be well-versed in common abbreviations, know how transaction amounts can affect the category, perform investigative research on novel entity names, and more.
Unless you have a lot of experience, it’s difficult to label these! That’s why having data labelers with the right skills is essential to creating quality datasets.
For this project, we built a team of Surgers with accounting and finance backgrounds, who've worked on our other financial categorization data labeling projects.
Data Labeling Interface
Here's a peek at our data labeling UI. Our platform makes it fast to create new data annotation and data collection jobs, whether through our API or our WYSIWYG editor.
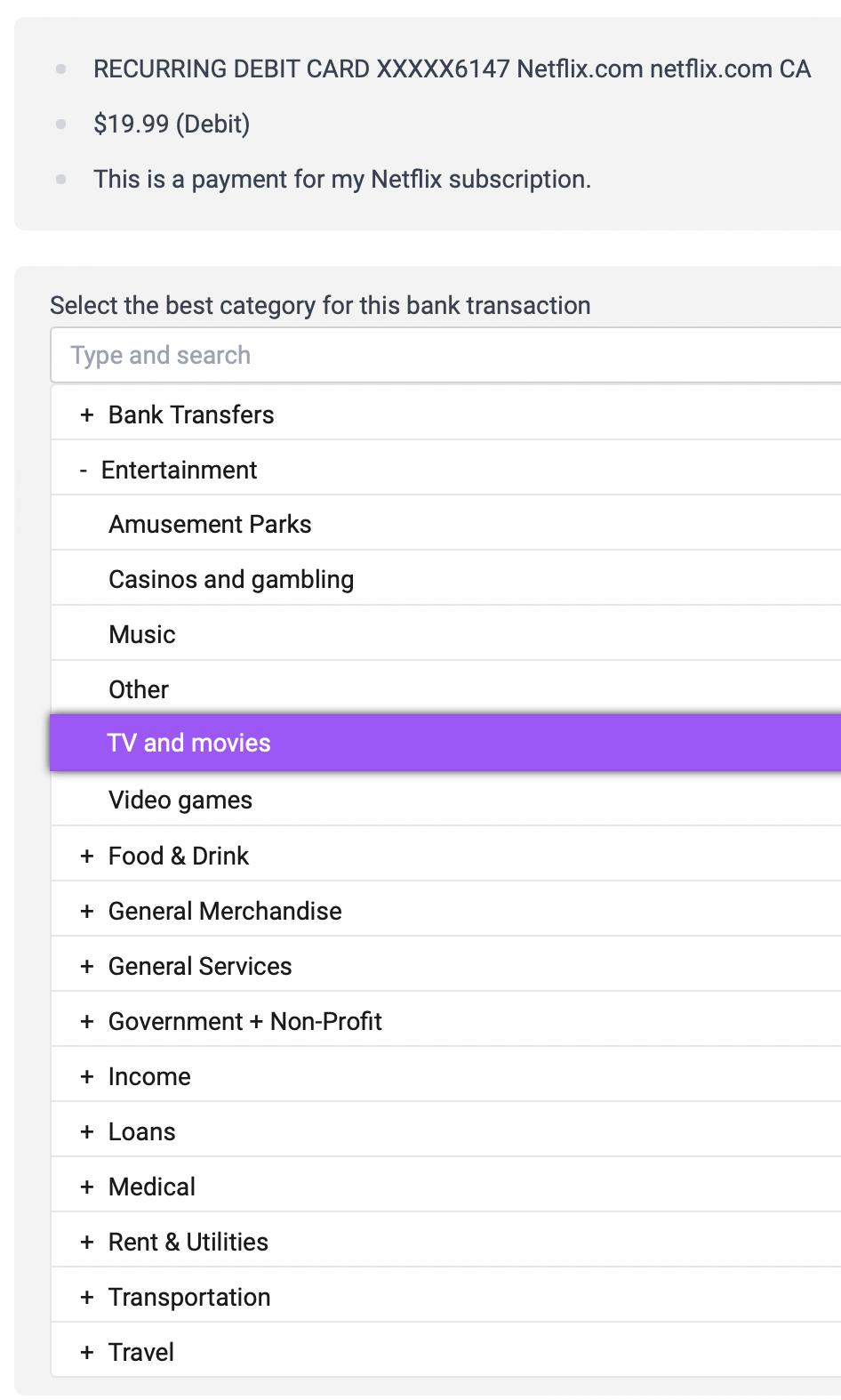
More Surge AI Datasets
Want to build a custom financial transactions dataset, or need help with other data labeling projects? Sign up and create a new labeling project in seconds, or reach out to team@surgehq.ai!
Interested in more data? Check out our other free datasets:
- Stock Market Sentiment Analysis
- Crypto Sentiment on Reddit
- Or browse the full list here!
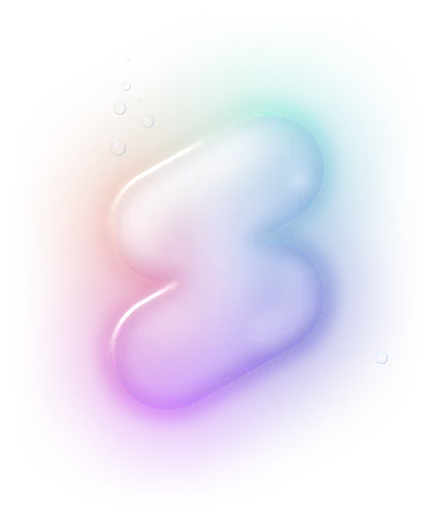
Data Labeling 2.0 for Rich, Creative AI
Superintelligent AI, meet your human teachers. Our data labeling platform is designed from the ground up to train the next generation of AI — whether it’s systems that can code in Python, summarize poetry, or detect the subtleties of toxic speech. Use our powerful data labeling workforce and tools to build the rich, human-powered datasets you need today.