
Misinformation is a thorny issue for social media platforms. On the one hand, platforms want to allow the spread of new ideas – who knows where the next spark of genius will arise? On the other hand, the spread of misinformation can be dangerous.
It's a tricky balance! Consider, for example, how the CDC called face masks ineffective, or how Twitter blocked tweets about Hunter Biden’s laptop.
We help leading social media companies and nonprofits around the world fact-check and study misinformation. By building teams of Surgers who are politically balanced (for example, by making sure that every post is reviewed by both left-leaning and right-leaning reviewers) and well-versed in the dark corners of the Internet, customers have found that our misinformation datasets are higher-quality than what even professional fact-checkers produce!
To that end, we're releasing a dataset of Facebook misinformation. It contains 500 posts that Surgers across the political spectrum found misinformative.
Explore and download the Facebook misinformation dataset here!
Facebook Misinformation Examples
Here are a few misinformation examples from the dataset.

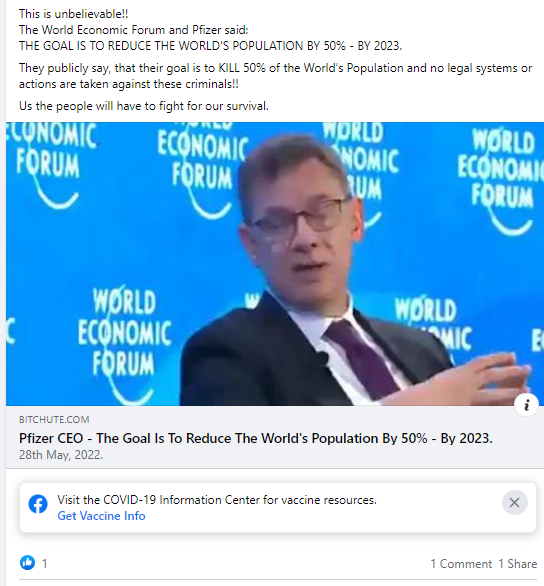


Misinformation Labeling Guidelines
One of the difficulties faced by companies who label misinformation – whether to measure its prevalence, or to train machine learning models to detect it – is that their guidelines often contain subtle errors.
Why does this happen? Guidelines are often written by people who don’t see many examples of misinformation in the real world. Thus, their labeling guidelines are based on idealized thoughts based on examples that they hear about in the news, rather than real world examples in the wild.
For example, we’ve seen misinformation guidelines that ask labelers to only label posts that contain statements of facts. In theory, this makes sense – you don’t want to label opinions as misinformation.
However, what about deepfake videos – for example, posts that put fake audio into the President’s mouth? Or posts that take real content, but splice them together in misleading ways? These may not contain explicit “statements of fact” per se, but likely fall into the bucket of content a Misinformation team should want to capture.
This may seem like an easily resolvable problem, but the problem is that an operations manager designing the labeling task may ask “Does this post contain a statement of fact?” as the first question, and skip the rest of the questions if the answer is No. Operations managers at larger companies often don’t take time to label examples themselves or listen to feedback from their labelers, and in practice, the issue may go undetected for months or years. It’s crucial to have good labeling infrastructure and best practices!
Creating good labeling guidelines is hard. That's why we partner with misinformation researchers, and comb through hundreds of thousands of posts to understand the nuances in order to create ours. If you need help with your labeling guidelines, we’re always happy to help – just reach out to team@surgehq.ai.
More Surge AI Datasets
Want to build a custom social media misinformation dataset, or need help with other data labeling or content moderation projects? Sign up and create a new labeling project in seconds, or reach out to team@surgehq.ai for a fully managed end-to-end labeling service.
Interested in more data? Check out our other free datasets and blog posts:

Data Labeling 2.0 for Rich, Creative AI
Superintelligent AI, meet your human teachers. Our data labeling platform is designed from the ground up to train the next generation of AI — whether it’s systems that can code in Python, summarize poetry, or detect the subtleties of toxic speech. Use our powerful data labeling workforce and tools to build the rich, human-powered datasets you need today.