During the Gamestop saga of January 2021, the WallStreetBets subreddit successfully short squeezed Gamestop’s stock, leading to a $7B loss for Melvin Capital and memes galore.
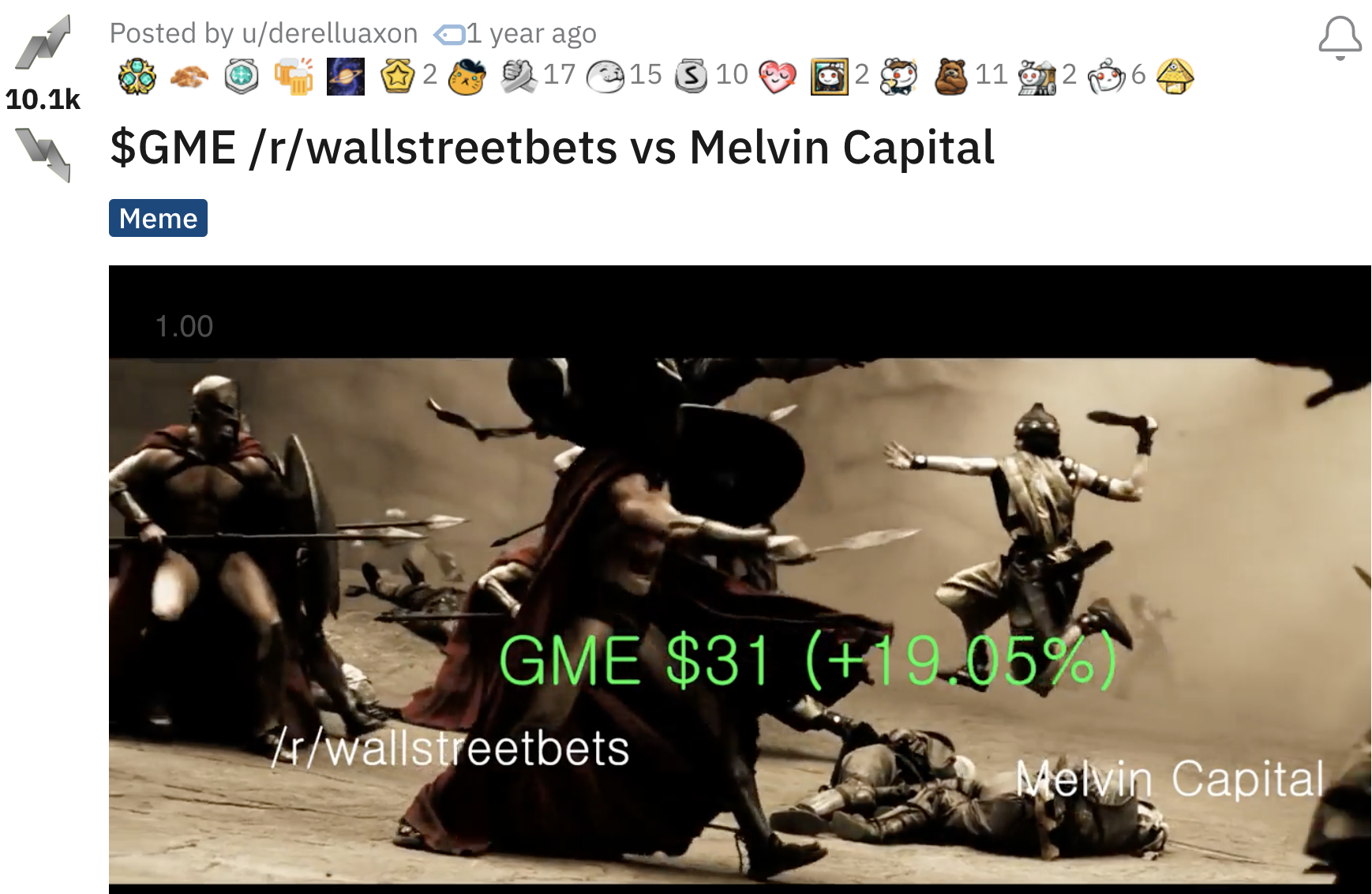
Too bad Melvin didn’t have a crack ML team monitoring social media!
To help others explore social media's influence on the stock market — and avoid Melvin Capital's fate — we created a dataset of social media conversations about public stocks, labeled with sentiment.
Have ideas for other datasets you’d like us to release? Give us a shout on Twitter at @HelloSurgeAI.
The Stock Sentiment Analysis Dataset
The dataset contains 1000 social media discussions of publicly traded stocks, with a Positive or Negative sentiment associated with each. Some of the sentiment is unequivocal; others are much trickier for models to classify correctly, since their sentiment is masked by sarcasm and trading-specific language.
Here are some examples. Are you confident that your classifiers can label them appropriately?
For example, can your model detect the sarcasm, and realize that this message is actually Positive towards $SNOW?
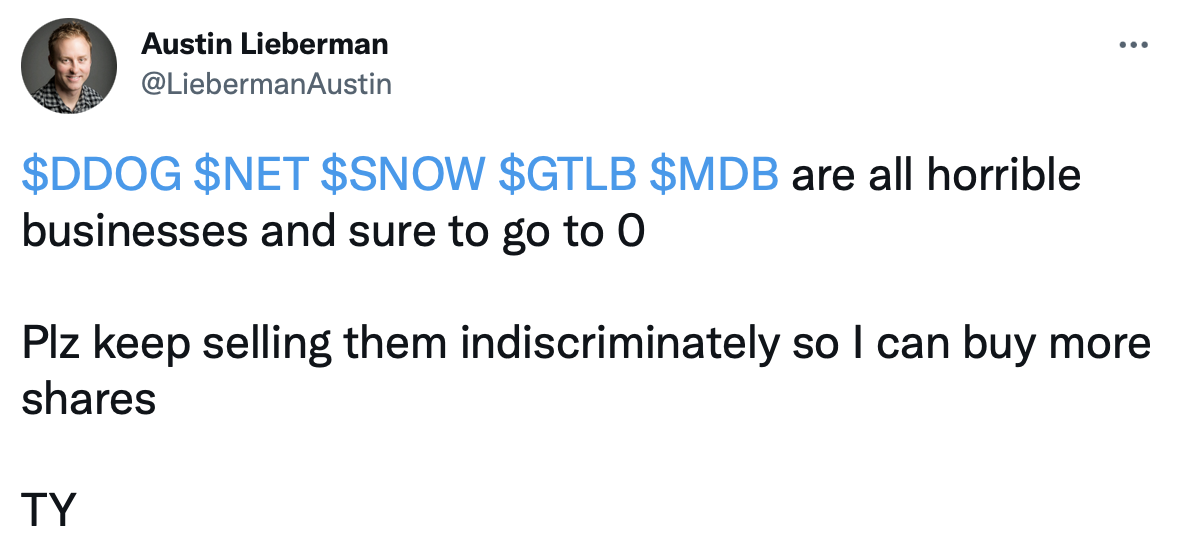
How would it classify this tweet? It's overall Positive in sentiment towards Ariose Capital Management 13F, but mentions that they've exited $NVDA. Can your model parse the structure?
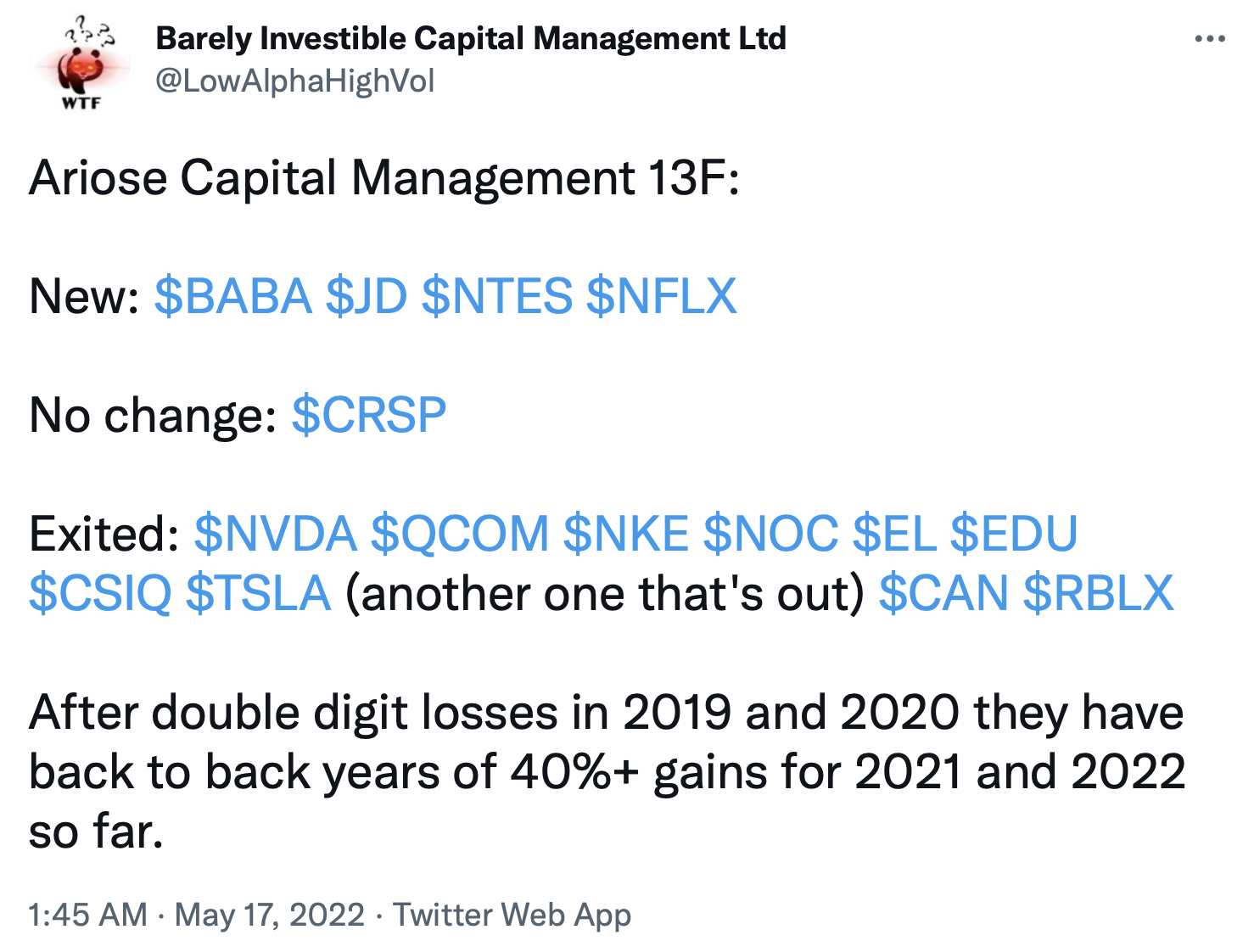
Many off-the-shelf sentiment analysis classifiers mistakenly classify any profanity (even obscured profanity, like fack) as negative in sentiment. But profanity isn't always a bad sign!
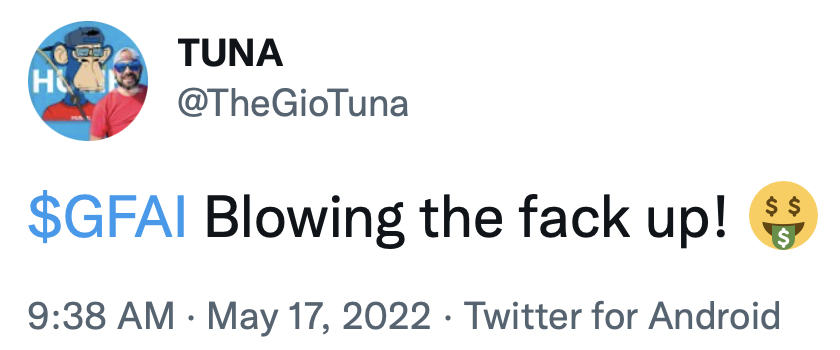
How would your sentiment classifier perform? Download the dataset and try it out here: https://github.com/surge-ai/stock-sentiment
How We Labeled the Dataset
As seen from the examples above, labeling the sentiment of a message towards a particular stock can be surprisingly tricky. And often you need domain knowledge: if someone is talking about a buying a put or a short squeeze, is that positive or negative? Unless you’re familiar with these financial terms, you may not know how to label it.
Having data labelers with the right skills is essential to creating quality datasets. For this project we used a team of Surgers with financial backgrounds who are also heavy social media users, who've worked on our other financial categorization and social media data labeling projects.
Here's a peek as well at our labeling UI as well. Our platform makes it easy to create new labeling jobs, whether through our API or our WYSIWYG editor.
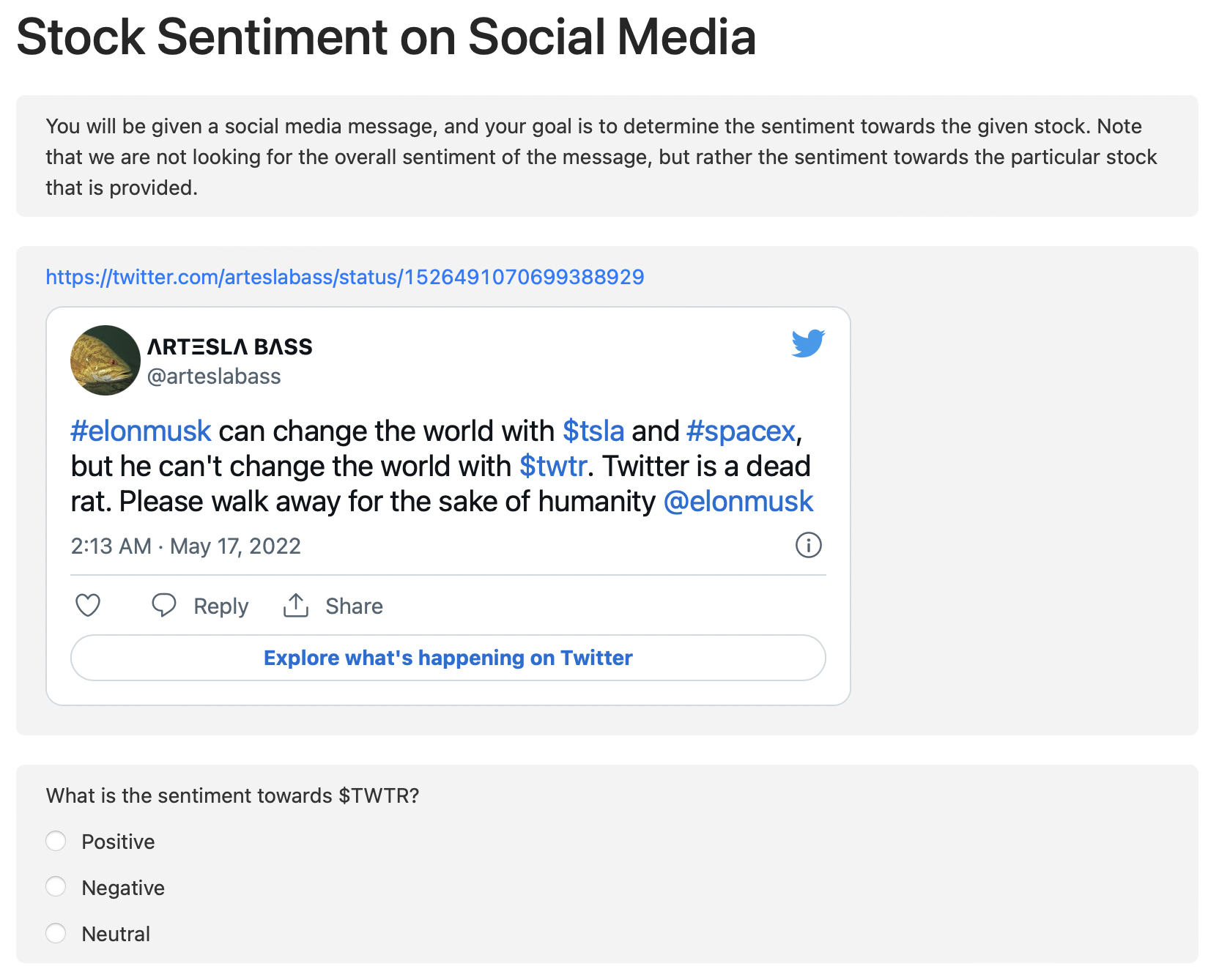
Want to create a custom financial dataset that will take you to the moon? Sign up and create a new labeling project in seconds, or reach out to us for help at hello@surgehq.ai!
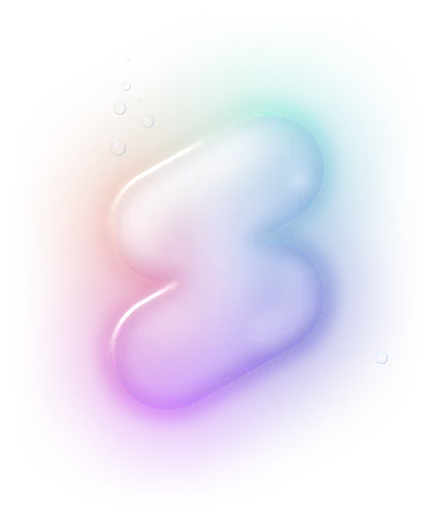
Data Labeling 2.0 for Rich, Creative AI
Superintelligent AI, meet your human teachers. Our data labeling platform is designed from the ground up to train the next generation of AI — whether it’s systems that can code in Python, summarize poetry, or detect the subtleties of toxic speech. Use our powerful data labeling workforce and tools to build the rich, human-powered datasets you need today.