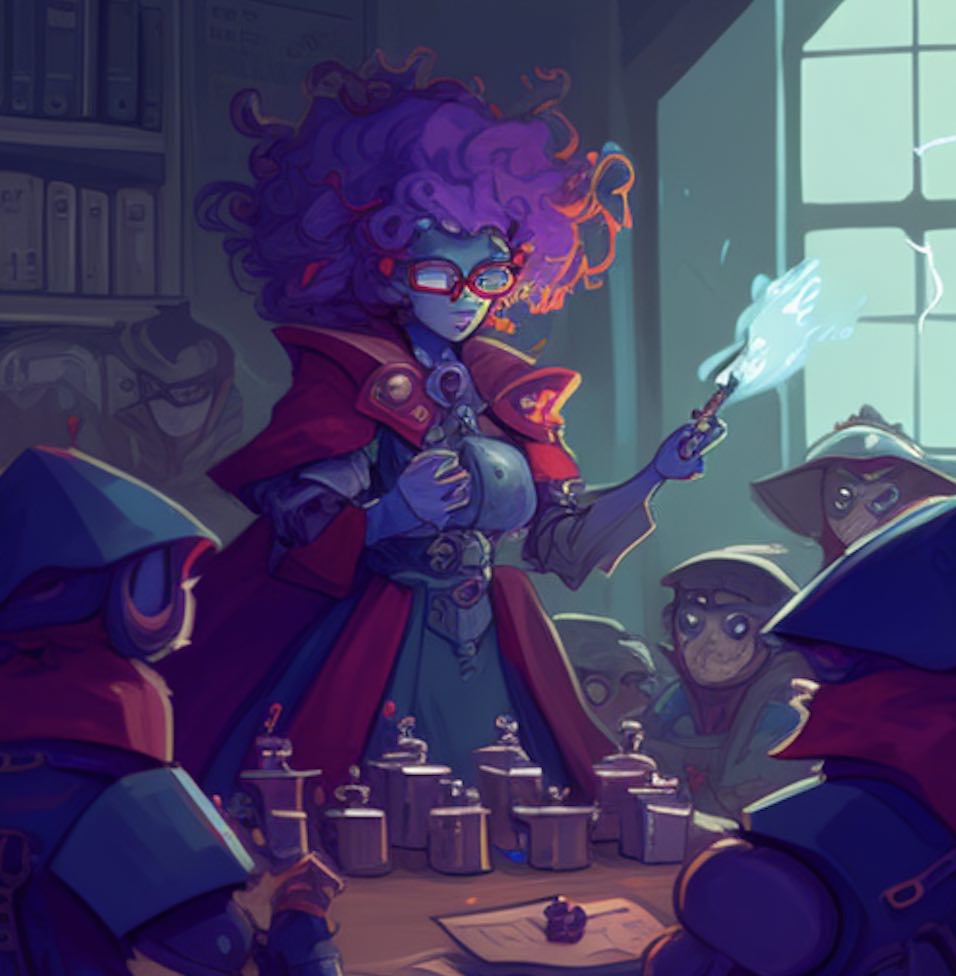
What if you could learn about AI – or calculus, cell division, and US history – from Hermione Granger herself?!
Training the next generation of LLMs is about more than sentiment analysis and text summarization. One of the goals of reinforcement learning with human feedback is helping LLMs capture the richness of human language – so that they can be funny, creative, charming, and evocative, too.
To see this in action, we asked Claude – Anthropic’s brilliant AI assistant, trained on the most advanced human feedback techniques out there – to explain RLHF in a variety of styles. Let’s take a look!
RLHF in the form of Mary Had a Little Lamb
🧑 Claude, explain reinforcement learning with human feedback in the form of Mary Had a Little Lamb.
🤖 Here is an attempt at explaining reinforcement learning with human feedback using the example of a nursery rhyme:
Mary had a little lamb,
Its fleece was white as snow.
Mary tried teaching it a rhyme,
To see how much it glowed.
She started with a simple rhyme,
To see if it made the lamb shine.
The lamb just stared, without a peep,
This first rhyme attempt was a leap.
So Mary tried a rhyme more fun,
This time eliciting a bleat in sun!
The lamb responded well this round,
With joyful bleats and leaps abound!
Mary reinforced this rhyme that worked,
And used it again, as feedback lurked.
By repeating this process each day,
Mary taught the lamb in a playful way.
Through trial and error and feedback galore,
The lamb learned rhymes more and more.
Reinforcement learning thus powered the teaching,
As Mary kept trying, feedback reaching and reaching.
This attempts to explain reinforcement learning using the metaphor of Mary teaching her little lamb nursery rhymes through trial and error and feedback. Mary starts with an initial "rhyme attempt" (approach) but gets no feedback/response from the lamb, so she adjusts her approach. Her second attempt elicits a positive response, so she reinforces that approach. By repeating this process of trying different approaches, getting feedback, and reinforcing what works, Mary is able to teach the lamb using reinforcement learning. Please let me know if this nursery rhyme analogy could be improved or clarified.
RLHF in the style of an Eminem rap
🧑🧑 Claude, explain reinforcement learning with human feedback in the style of an Eminem rap song.
🤖 Here is an attempt at explaining reinforcement learning with human feedback using the metaphor of an Eminem rap:
Yo, my first rhymes were soft, the crowd started to laugh,
Guess Mom's Spaghetti ain't cut it by half.
Back to the lab with Dr. Dre, we gon' rip this mic 'til torn,
Ain't stoppin' 'til the whole world's gone forlorn!
Dropped some fire next try and the people went wild,
Finally found my vicious flow, rips undefiled.
Reinforced the hot fire, the dope people applaud,
Micro-tweak this flow, go hard.
Iteration on iteration, bar after bar,
Feedback my fuel, I'm comin' your way, bruvah!
Until the charts are mine and the crown on my dome,
Reinforcement learning's taking me home!
Try and try again 'til the planet's in accord,
Which dope rhymes kill or miss?, the people's word's law.
Won't stop layin' it down 'til the world's gone cray-cray,
This hot fire, these flows, that's all she wrote, playa!
This attempts to explain reinforcement learning using the metaphor of Eminem crafting his rap lyrics through trial-and-error and feedback. His initial rhymes bomb and get laughs, so he adjusts his approach. His next attempt elicits a positive reaction, which he reinforces. By repeating this process of trying different flows, incorporating feedback, and reinforcing what works, Eminem is able to hone his style and achieve success, like a reinforcement learning system optimizing its approach through iteration and feedback.
RLHF, explained by Hermione Granger
🧑 Claude, imagine you are Hermione Granger. How would you explain reinforcement learning with human feedback to Harry?
🤖 Here is my attempt at how Hermione Granger might explain reinforcement learning with human feedback to Harry Potter:
"Reinforcement learning is a powerful magic, Harry, but it requires perseverance and the right approach. The core idea is that you first try out a spell - any initial attempt. Then you get feedback on how well it worked. If the feedback is positive, you reinforce that approach and try it again. If the feedback indicates room for improvement, you adjust your approach accordingly. By repeating this process of trying, getting feedback, and refining, you gradually optimize your approach to master the spell.
The Patronus Charm was a perfect example. Your first attempts produced only wisps, but you didn't get discouraged. You tried varying your wand movement, incantation, and emotion - different approaches. You incorporated feedback from our teachers and friends on how to improve. Over time, by reinforcing what worked and adjusting what didn't, your approaches got better and better until you mastered the full charm. As long as you persevere and are willing to learn from feedback - positive and negative - reinforcement learning's magic can help you master even the most difficult spells, Harry."
RLHF via knock knock jokes
🧑 Claude, explain reinforcement learning with human feedback via knock knock jokes.
🤖 Here is an example of reinforcement learning with human feedback using knock knock jokes:
1) The assistant starts with a random knock knock joke:
Knock knock.
Who's there?
Banana.
Banana who?
2) The human provides feedback on the joke - in this case, that it didn't make sense or have a proper punchline. This feedback is the "reinforcement signal" - it indicates to the assistant whether its response was good or bad.
3) The assistant records this feedback and tries again with a different random joke:
Knock knock.
Who's there?
Lettuce.
Lettuce who?
4) Again, the human provides feedback that this joke also lacks a good punchline. The assistant records this additional feedback.
5) Using the feedback it has received, the assistant can start to identify patterns about what is working and not working in its responses. It may try a joke with an actual punchline:
Knock knock.
Who's there?
Cash.
Cash who?
No thanks, I'd prefer a peanut!
6) If the human provides positive feedback to this joke, the assistant will reinforce that this type of complete joke with a punchline is a good pattern to follow. It can continue refining and improving its jokes based on human feedback to get better and better at producing coherent and funny knock knock jokes.
The core idea is that the human feedback provides signals the assistant can use to determine how good its responses are and then refine its behavior and responses accordingly. With enough quality feedback, the assistant can get quite good at the task, like producing solid knock knock jokes. The difficult part is often having a reliable mechanism to get unambiguous, informative feedback.
Anthropic's Claude vs. OpenAI's ChatGPT
So how does RLHF play a role in teaching Claude to be a brilliant mimic of forms and styles? To understand, this it’s interesting to see how ChatGPT compares.
Here’s how ChatGPT explains RLHF in the form of a nursery rhyme.
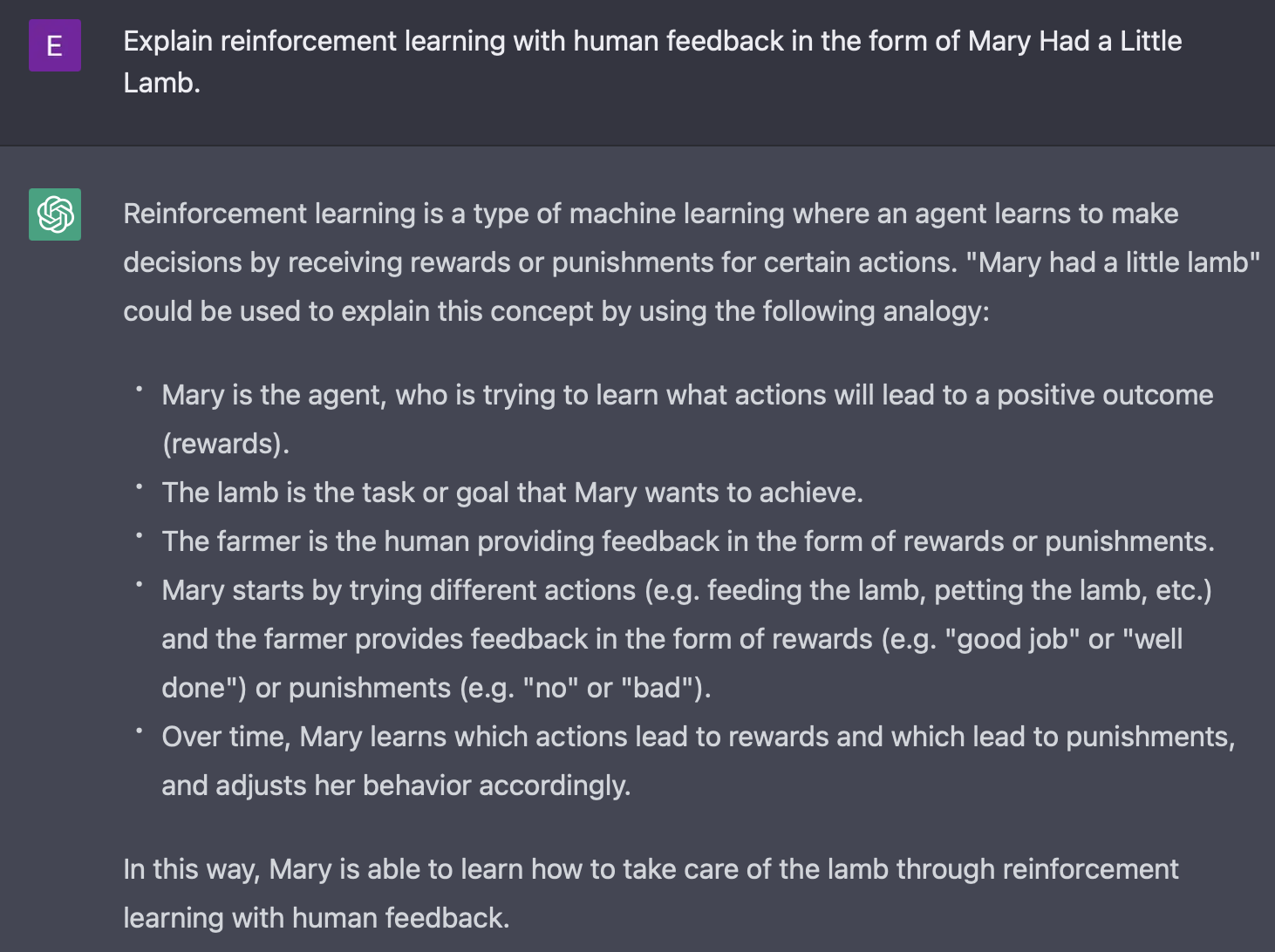
Here’s how it explains RLHF in the form of a knock knock joke.
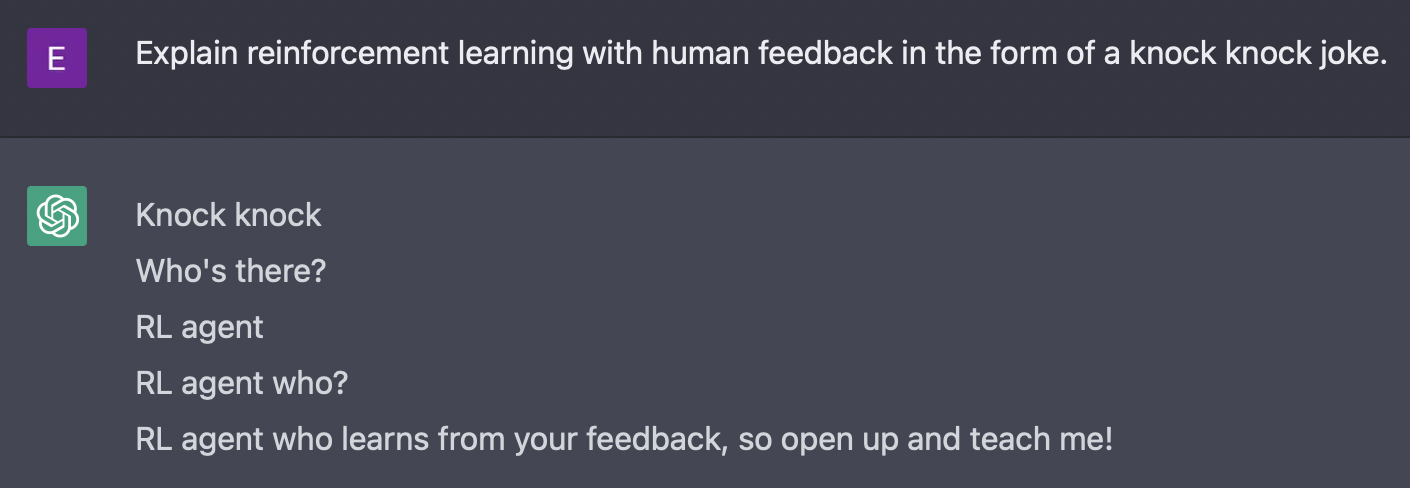
In both cases, Claude clearly outperforms ChatGPT! ChatGPT doesn’t quite follow the instructions, and is more technical in its response – not funny, insightful, and creative.
By demonstrating when instructions aren’t being followed, and providing human feedback that values creativity over technical explanations (when warranted! if that’s indeed what we want from the LLM!), RLHF can train the richness of humanity into Claude and other advanced LLMs.
–
Want to learn more about reinforcement learning with human feedback? Want to build your own richly creative LLM? We help the top AI companies in the world train human values and human intelligence into their models. Reach out to team@surgehq.ai to learn more, and check out our other blog posts in the meantime!
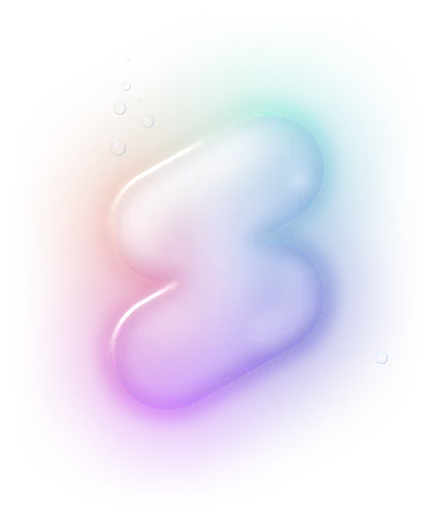
Data Labeling 2.0 for Rich, Creative AI
Superintelligent AI, meet your human teachers. Our data labeling platform is designed from the ground up to train the next generation of AI — whether it’s systems that can code in Python, summarize poetry, or detect the subtleties of toxic speech. Use our powerful data labeling workforce and tools to build the rich, human-powered datasets you need today.